๐ธ๏ธ A Simple Proxy for Azure and OpenAI raised our GPT4 TPM limit by 24x
William Zeng - October 26th, 2023
OpenAI API rate limits are restrictive, with a top rate limit of 20K TPM for GPT4.
Even the most basic AI Agents make 3+ calls per request, and to effectively plan theyโll need to use RAG(retrieval augmented generation) (opens in a new tab). A minimally useful request should require an input of at least 500
tokens(~375 words ~ 2 paragraphs) worth of text, and generate roughly 125
tokens(half a paragraph?) of output.
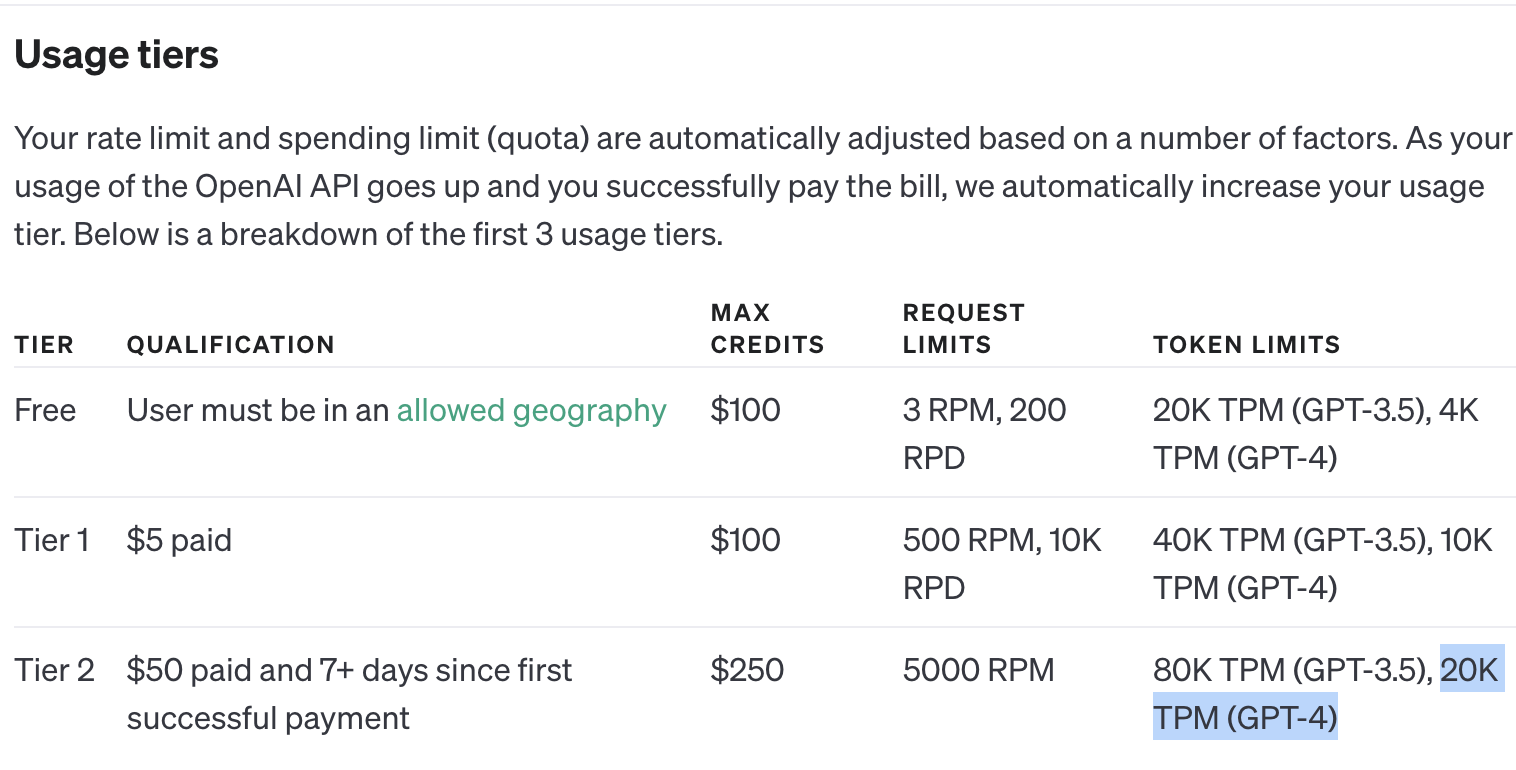
Over a single request this equates to 1,875(3 * 625)
tokens, hitting the token limit with 10.67
requests/min.
For reference, a Sweep ticket makes 20
GPT4 calls consuming an average of 2000
tokens and a total usage of 40,000
tokens.
This leads to a "Sweep" request limit of 0.5
requests/min, needing 2 minutes to finish a single request.
Thereโs a solution though.
Microsoft Azure East US has a 20K TPM limit for us.
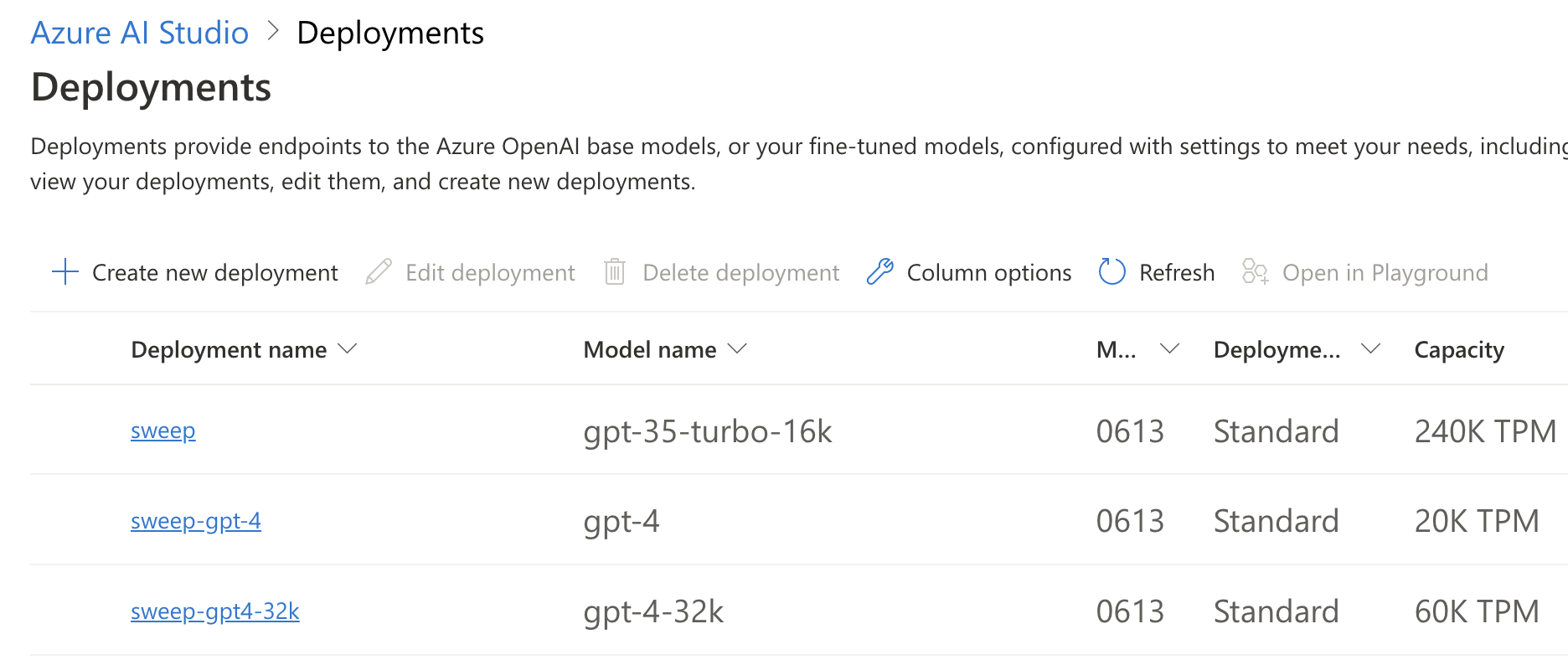
We can use a proxy like(https://github.com/diemus/azure-openai-proxy (opens in a new tab)) to load the balance between OpenAI and Azure, bringing our total TPM limit from 20k โ 40k.
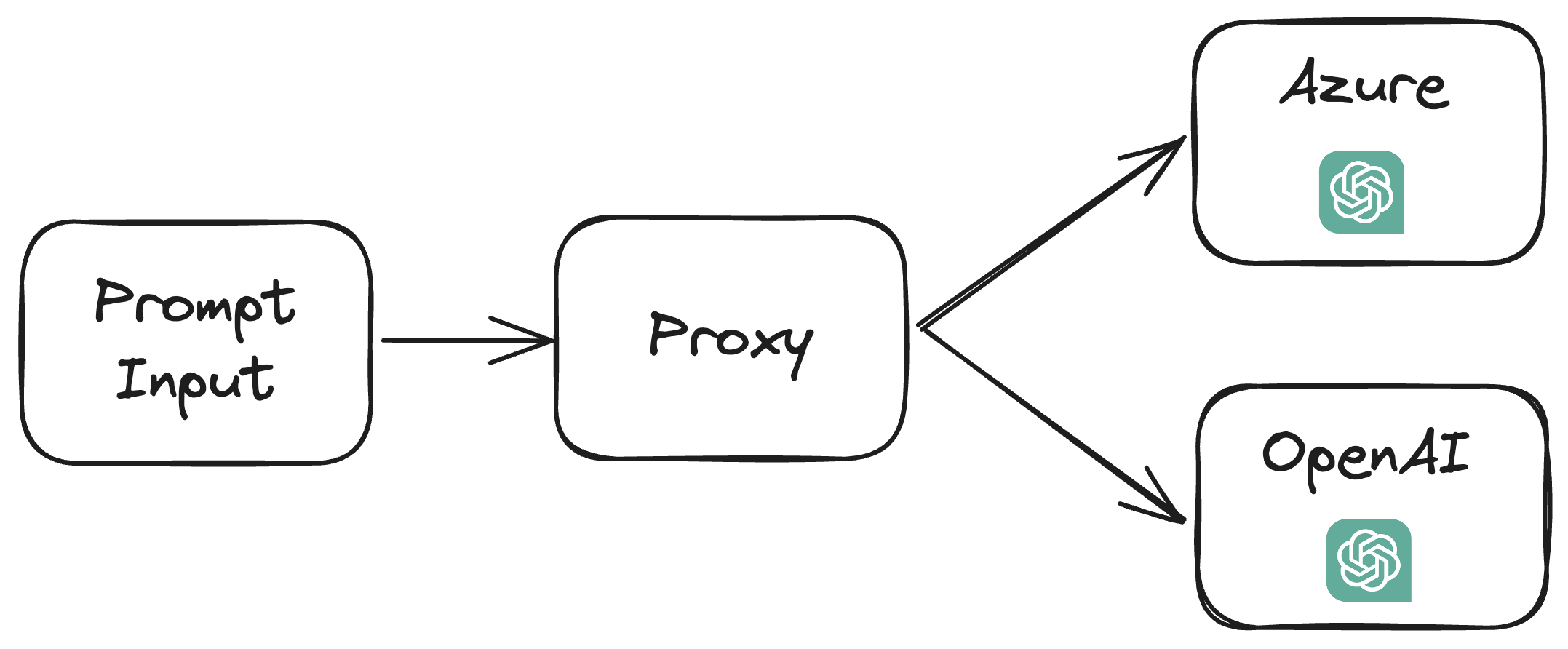
This helps, but it only brings us up to 40k total TPM. Even better, we can balance between multiple Azure regions to further increase our rate limit.
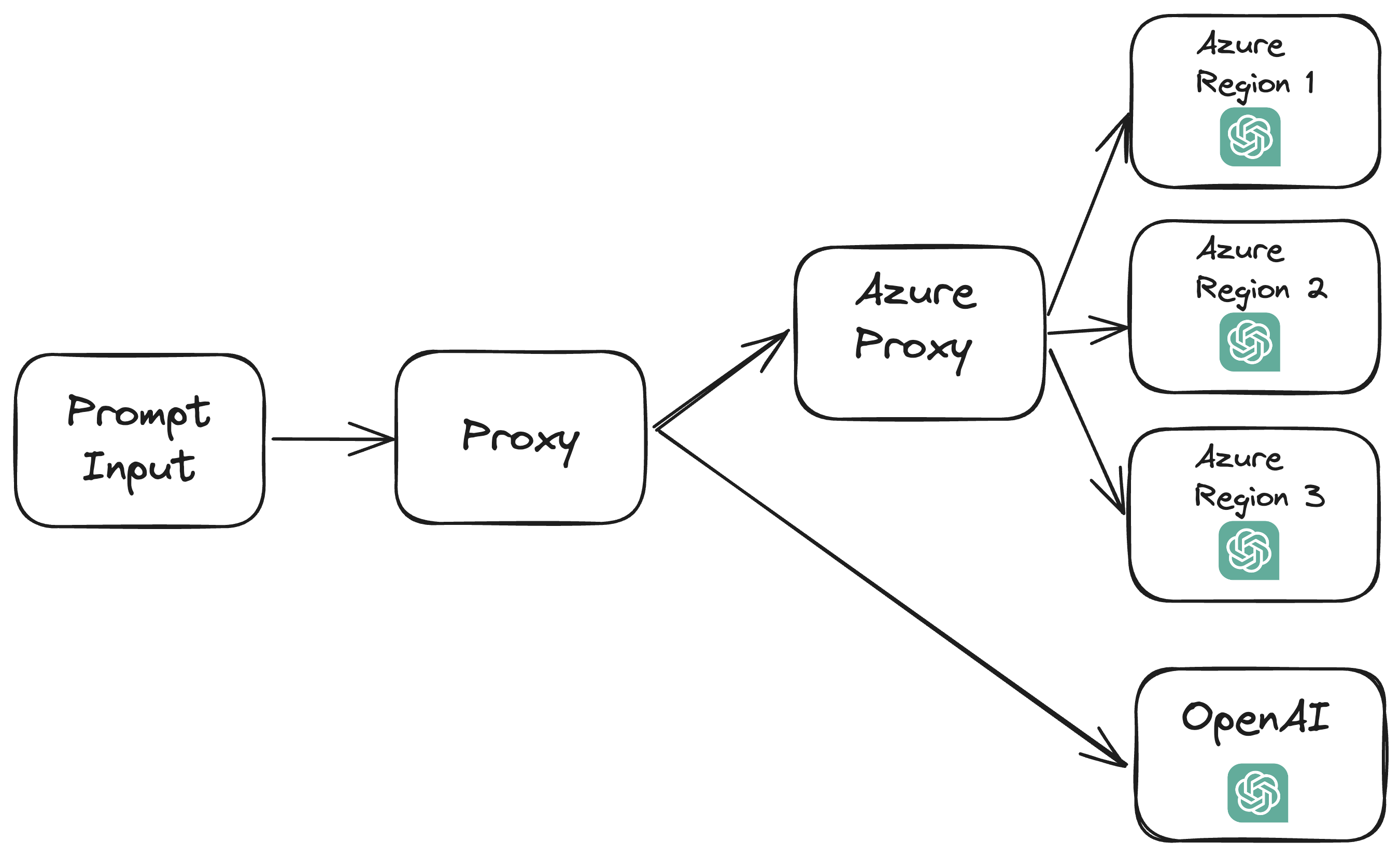
Hereโs a table of the rate limits we found on each region:
Azure Region | GPT-4 Rate Limit(TPM) |
---|---|
East Canada | 100K |
Japan East | 100K |
East US 2 | 100K |
UK South | 100K |
Australia East | 40K |
Switzerland North | 40K (dynamic) |
East US | 20K |
France Central | 20K |
Sweden Central | NA |
West Europe | NA |
North Central US | NA |
Total | 480K/520K(dynamic) |
Using all of the Azure regions, we were able to increase our TPM rate limit from 20k
โ 40k
(2x increase!) โ 480K
(24x increase!!)
Hereโs a graph of our OpenAI usage before/after this change:
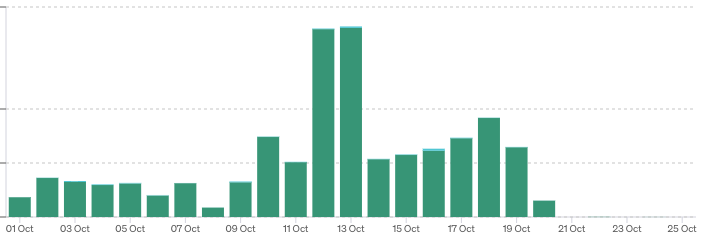
Here's the code:
Sweep's OpenAI Proxy
import random
import openai
from loguru import logger
from sweepai.config.server import (
AZURE_API_KEY,
MULTI_REGION_CONFIG,
OPENAI_API_BASE,
OPENAI_API_ENGINE_GPT4,
OPENAI_API_ENGINE_GPT4_32K,
OPENAI_API_ENGINE_GPT35,
OPENAI_API_KEY,
OPENAI_API_TYPE,
OPENAI_API_VERSION,
)
class OpenAIProxy:
def __init__(self):
pass
def call_openai(self, model, messages, max_tokens, temperature) -> str:
try:
engine = None
if (
model == "gpt-3.5-turbo-16k"
or model == "gpt-3.5-turbo-16k-0613"
and OPENAI_API_ENGINE_GPT35 is not None
):
engine = OPENAI_API_ENGINE_GPT35
elif (
model == "gpt-4"
or model == "gpt-4-0613"
and OPENAI_API_ENGINE_GPT4 is not None
):
engine = OPENAI_API_ENGINE_GPT4
elif (
model == "gpt-4-32k"
or model == "gpt-4-32k-0613"
and OPENAI_API_ENGINE_GPT4_32K is not None
):
engine = OPENAI_API_ENGINE_GPT4_32K
if OPENAI_API_TYPE is None or engine is None:
openai.api_key = OPENAI_API_KEY
openai.api_base = "https://api.openai.com/v1"
openai.api_version = None
openai.api_type = "open_ai"
logger.info(f"Calling {model} on OpenAI.")
response = openai.ChatCompletion.create(
model=model,
messages=messages,
max_tokens=max_tokens,
temperature=temperature,
)
return response["choices"][0].message.content
# validity checks for MULTI_REGION_CONFIG
if (
MULTI_REGION_CONFIG is None
or not isinstance(MULTI_REGION_CONFIG, list)
or len(MULTI_REGION_CONFIG) == 0
or not isinstance(MULTI_REGION_CONFIG[0], list)
):
logger.info(
f"Calling {model} with engine {engine} on Azure url {OPENAI_API_BASE}."
)
openai.api_type = OPENAI_API_TYPE
openai.api_base = OPENAI_API_BASE
openai.api_version = OPENAI_API_VERSION
openai.api_key = AZURE_API_KEY
response = openai.ChatCompletion.create(
engine=engine,
model=model,
messages=messages,
max_tokens=max_tokens,
temperature=temperature,
)
return response["choices"][0].message.content
# multi region config is a list of tuples of (region_url, api_key)
# we will try each region in order until we get a response
# randomize the order of the list
SHUFFLED_MULTI_REGION_CONFIG = random.sample(
MULTI_REGION_CONFIG, len(MULTI_REGION_CONFIG)
)
for region_url, api_key in SHUFFLED_MULTI_REGION_CONFIG:
try:
logger.info(
f"Calling {model} with engine {engine} on Azure url {region_url}."
)
openai.api_key = api_key
openai.api_base = region_url
openai.api_version = OPENAI_API_VERSION
openai.api_type = OPENAI_API_TYPE
response = openai.ChatCompletion.create(
engine=engine,
model=model,
messages=messages,
max_tokens=max_tokens,
temperature=temperature,
)
return response["choices"][0].message.content
except SystemExit:
raise SystemExit
except Exception as e:
logger.exception(f"Error calling {region_url}: {e}")
raise Exception("No Azure regions available")
except SystemExit:
raise SystemExit
except Exception as e:
if OPENAI_API_KEY:
try:
openai.api_key = OPENAI_API_KEY
openai.api_base = "https://api.openai.com/v1"
openai.api_version = None
openai.api_type = "open_ai"
logger.info(f"Calling {model} with OpenAI.")
response = openai.ChatCompletion.create(
model=model,
messages=messages,
max_tokens=max_tokens,
temperature=temperature,
)
return response["choices"][0].message.content
except SystemExit:
raise SystemExit
except Exception as _e:
logger.error(f"OpenAI API Key found but error: {_e}")
logger.error(f"OpenAI API Key not found and Azure Error: {e}")
raise e
MULTI_REGION_CONFIG
is a list of tuples of (region_url, api_key)
that we randomize over, and the other envvars are standard strings from OpenAI/Azure. Another requirement with our proxy is that each of the models(GPT3.5, GPT4, GPT4-32K) must have consistent naming across regions.
We have some potential improvements to our proxy:
- We randomize between the different Azure regions uniformly, so this could be improved by weighted randomization.
- We could decrease our expected latency further by keeping track of which regions have capacity.
- We could optimize further by trying to estimate the load on each region. We can perform a smart optimization by computing a simple function like:
estimated_latency = mean_over_regions((input_tokens + output_tokens) / latency_per_token_estimate)
estimated_load = estimated_latency - true_latency
- and then optimize the region-level randomization by negatively weighting the
estimated_load
Here's a link to our production code:
https://github.com/sweepai/sweep/blob/main/sweepai/utils/openai_proxy.py (opens in a new tab)
If you're looking for a more advanced version of this, check out LiteLLM's load balancer (opens in a new tab).
If you're interested in using Sweep/have questions about how we use LLMs in production, reach out at https://discord.gg/sweep (opens in a new tab)!